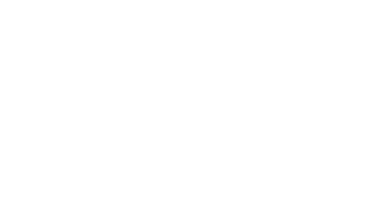
- Start date
- Duration
- Format
- Language
- 17 Sept 2024
- 5 days
- Online
- Italian
Fornire gli strumenti per (ri)disegnare la roadmap di adozione e sviluppo dell’AI in azienda bilanciando strategia, elementi tecnologici, organizzativi e di contesto.
The news we read in the papers every day reminds us that in this age of hyper-connectivity, the destiny of any given business is more uncertain than ever from a competitive, technological, and regulatory standpoint. These are particularly turbulent times - we see companies coming out of nowhere and demolishing consolidated sectors, setting the stock markets on fire. But often sooner or later they fade into obscurity again. Sometimes we find giants tramping onto the scene, seemingly unstoppable, expanding their domains to more and more markets every day.
A hyper-connected world creates the conditions that make it easy for new enterprises to emerge. Just think: (1) Digital businesses are more scalable and their strategies more fluid. (2) There are fewer barriers to entry in terms of distribution channels. (3) Fewer investments are needed for typically “asset light” models, which among other things, are similar in that they have (4) easier access to financing, and fewer obstacles on the path to brand awareness. (5) Case in point is the lightning speed with which brilliant ideas start trending online in the age of social media. Added to all this, in particular for younger users, is (6) low behavioral inertia, or to put it another way, a high propensity to constantly try out new things, new apps, new social media, and so forth. This behavioral trait is called “novelty seeking” which, along with “variety seeking,” generates economics of variety on the demand side and a constant stream of new opportunities on the supply side.
But these same factors make defending competitive positions far more complex, even for companies that strive for continual innovation. The reasons for this are essentially two, both with common roots but different implications. First, the revolution that comes after mine will happen faster than before, accelerating the so-called “clock speed” of competitive cycles, thanks to the digital paradigm which serves as facilitator. As an example of this, consider how the Yahoo! revolution was quickly swept aside by what came later: Google. Or how TikTok became an overnight sensation among very young users, usurping the platforms of Mark Zucherberg, initiator and icon of the previous social media revolution.
The second factor that heightens the vulnerability of competitive positions relates back to the six conditions listed above, which in a hyper-connected world, make it easier to imitate the first mover. For examples here, just look at the trials and tribulations of Uber or eBay in China: in a few short years, the two American innovators had to give way to local imitators, Didi and Taobao respectively. This should serve as a reminder that the advantage of network economies in the digital age is sometimes misunderstood and overestimated, in particular when users can access several platforms simultaneously, which is what happens with food delivery or car sharing.
Naturally this doesn’t mean that first-mover advantages have disappeared. Quite the contrary. Some incumbents (just a few) can enjoy greater benefits than before, but only when additional conditions coexist. Specifically, tying into what we mentioned above, it can’t be cheap for a user to access more than one ecosystem. For instance, it’s very easy to use both Just Eat and Deliveroo, but it’s far more expensive to own both an iOS and an Android cell phone. What’s more, when there are network externalities, they have to impact core aspects of value, not simply peripheral value dimensions.
The same is true for data, with all the acclaimed advantages - which at times are overestimated too. Let’s try to explain with two examples, one that mirrors the other. On one hand, let’s imagine a company that uses machine leaning algorithms to help doctors do better at interpreting the results of diagnostic imaging. On the other, we have a car manufacturer that utilizes data from its vehicles to offer customers a traffic prediction service. In the first case, given the complexity of the output, there is no limit to the amount of historical data (and medium-term data as well) that will be useful to fine-tune the algorithm. What’s more, for a hospital the level of precision would be a key consideration in choosing a supplier, one that would represent the core benefit of the purchase. In this kind of context, learning economies for the first mover will offer a crucial advantage, as the core value of the offering can constantly be improved.
In contrast, going back to the example of the car manufacturer, we can reasonably assume that a certain number of vehicles in a city will generate enough data to make accurate predictions on traffic. Below that number, my output will be less accurate, but above that threshold the marginal value of one additional datum would be negligible, because it wouldn’t add serviceable precision to my service. This would make the first-mover advantage much less defendable; in other words, it would make innovation easier to imitate. And that’s not all. Even if an optional like traffic prediction can enhance my value proposition, as far as choosing a car it’s hard to imagine that this optional would be the deciding factor for a customer, compensating for substantial disadvantages there might be in terms of the core components of the value proposition in question.
So as we can see from these example, data-driven models can provide a sustainable competitive advantage to first movers if the marginal benefit of a single datum does not decrease too quickly. If it does, the advantage of the innovator would tend to flatten out fast, from a certain point in time on, making imitation more simple and levelling the playing field. The quality of the algorithm outputs would be likely to converge in short order.
When the conditions we described above exist, that’s when the economies of scale and scope, and learning of the leader become really impressive, consolidating the dominant market positions over time. And that’s not all. The value of analytical and predictive abilities, digital infrastructures, the customer base and brand trust is transversal; in other words, it transcends the traditional industry borders (what’s called the “replicability of competitive advantage”). This will throw the doors wide open to inexorable expansion in many other contexts as well, further fueling the effects of scale, scope and learning.
Naturally, today’s managers must be fully aware of all these approaches. But as far as what we’ve discussed above, it’s equally critical to think about competitive dynamics, and to consider ways to properly regulate the market as well, so it always remains open to competition.