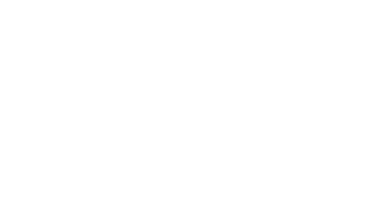
- Start Date
- Duration
- Format
- Language
- 14 Apr 2025
- 4,5 days
- Class
- Italian
Gestire in modo integrato e coerente una rete di vendita potenziando la capacità di prendere decisioni efficaci e sviluppando una visione strategica del sales management.
Management is a young discipline. It started in the 1920s and developed mostly after the Second World War, when the rise of large companies necessitated a better understanding of how to design and manage complex organizations, and make strategic decisions.
The problem with young disciplines is the lack of paradigms, which make it possible to build new understandings on solid beliefs and allow scholars and users to share a common language. In fact, although the study of organizations and firm strategies has achieved many milestones, it has now reached a critical juncture.
On the one hand, leading companies are looking for deeper insights than the descriptive positive analyses that populate management research today. On the other hand, AI is pushing for a different perspective.
This resurrects one of the original domains of management research, among the most important: decision-making. This is certainly an art, relying as it does on intuition, gut-feelings, and insights based on experience and tacit knowledge. But the same can be said of science, or even The Arts, such as music or painting. Scientists have intuitions, but they subject them to structured protocols, and then formulate clear theories and experiments so that other scientists or users can assess and understand them. Similarly, Bach composed Das Wohltemperirte Clavier to codify the paradigm of the 48 musical keys that laid out the basis of the music composed thereafter.
The blind spot in management research is that its best known and widely taught theoretical paradigms are Porter’s five forces and the Resource-Based View. Both produced in the past century, the first stresses the importance of competitors, customers, suppliers, new entrants, and substitute products; the second, the need to figure out the sources of non-imitable assets of an organization.
Stepping back, strategic management asks two questions:
This second question pushes the envelope and generates most of the value.
The good news is that analytical disciplines, coupled with AI, provide the foundations for understanding information acquisition and processing. Today’s overarching emphasis on data analysis is only a small part of the story. The more general problem is how to build and test reasoning models – theories – to work out how to use information to make decisions. First, theories identify the causes underlying successful business models or opportunities, and the perceived likelihood of the theory’s plausibility (prior beliefs). For instance, the founders of PayPal anticipated that they would have to address the question of security to unlock the world of electronic payments; Leonardo Del Vecchio foresaw that good design was critical to create a new market for fashion eyewear. Second, strategic decision-makers test their theories: Del Vecchio partnered with fashion houses to assess whether Luxottica could produce a good design, and the good design in turn generated demand.
The real problem is not only that the prompts that we feed into AI reasoning models make a difference, but that they make a bigger difference if the question is deeper. AI and an ordinary person would give similar answer to a simple question, but AI would give a better answer to a better posed, more sophisticated question.
This means strategic management must become a discipline of AI-prompts. This is not a disparaging statement – quite the opposite. We’re not talking about the prompts that we key into our chatbots, but “super-prompts” in the form of sophisticated agentic AI models. These build on theoretical frameworks and phenomenological knowledge of the problems, and on the underlying disciplines, such as mathematics, the theory (and not just the practice) of statistics, economics, cognitive sciences, and computer science. Scholars in strategic management need to acquire these competencies to maneuver the agentic AI models like Formula One drivers. They don’t develop the technologies their cars run on, but they know these technologies, and they can suggest changes to achieve higher driving performance.
In this spirit, the ION Management Science Lab at Bocconi University, which is devoted to moving the managerial sciences forward in the direction mapped out above, is set to host the 2nd Bayesian Entrepreneurship Conference on March 20-21, 2025, a pivotal event for strategy and entrepreneurship scholars. The keynotes and conference papers aim to build an interdisciplinary paradigm for connecting management science, education, business practices, and other organizations. The conference features as keynote speakers Itzhak Gilboa (HEC Paris), a leading authority on decision sciences, and Riccardo Zecchina (Bocconi University), a pioneer in machine learning and statistical physics.
Discover more:
Agrawal, A., Camuffo, A., Gambardella, A., Gans, J., Scott, E., & Stern, S. (2025), Bayesian Entrepreneurship, MIT Press.
Camuffo, A., Gambardella, A., & Pignataro, A. (2024). “Theory-Driven Strategic Management Decisions.” Strategy Science, 9(4), 382–396. DOI: https://doi.org/10.1287/stsc.2024.0173.
Camuffo, A., Gambardella, A., Messinese, D., Novelli, E., Paolucci, E., & Spina, C. (2024). “A scientific approach to entrepreneurial decision‐making: Large‐scale replication and extension.” Strategic Management Journal, 45(6), 1209–1237. DOI: https://doi.org/10.1002/smj.3580.