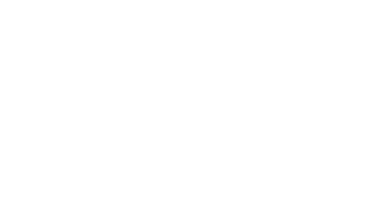
- Start date
- Duration
- Format
- Language
- 12 May 2024
- 3 days
- Class
- English
A journey through the digital revolution to learn how to capitalize on these enhancing technologies to gain competitive advantage in existing and new markets.
Data analysis technologies can make a substantial contribution to company growth by supporting management, operations and value generation mechanisms. But one essential ingredient is necessary to ensure that these advantages actually materialize: an effective data strategy.
For the past several years, businesses and institutions of every size and sector have been exposed to evident enticements from the constantly changing landscape of available technology. As regards how companies tend to utilize and integrate these technologies, a spectrum of options has gradually come into play, impacting value generation levers and operational mechanisms of work in different ways. In some cases, in fact, the approach to utilizing digital technologies consists of tactical integration to support and upgrade traditional processes. In other cases, instead, digital takes on the role of a transformative element in companies that choose to employ existing technological levers to evolve, transforming the mechanisms that the business deploys to generate and capture value on the market. The latter constitutes a true digital transformation approach which, depending on the context and the objectives, may or may not prove to be the best fit compared to a more tactical one.
Focusing on the companies that opt to take this transformative path, the evidence gleaned in recent years clearly shows that in order for a digital transformation to be a successful one, it must act on five dimensions:
The second vital contextual element pertains to the central role of data in pursuing many evolutionary and transformative paths. Indeed, many digital technologies are data-driven, creating a waterfall effect supporting decisions and processes, both from a tactical and a strategic perspective.
Using this framework as our starting point, the research we conducted explored five fundamental sectors (logistics, car manufacturing, retail, banking, and telecommunications), identifying and tracking the key role of data strategy as an element that can reconcile vision and strategy on one hand and levels of technological alignment on the other.
Data strategy, in fact, emerges as the sixth dimension of the digital transformation, proving to be crucial for companies like Vodafone. We selected the case history of this company to show how the dissemination of a data-based approach and culture is an important strategic factor to support many of its digital evolution objectives.
In the cases we analyzed for our research, various digital evolution projects clearly emerged with varying degrees of transformative objectives. For example:
The need to adopt a data strategy and deploy the right tools and methodologies to orchestrate data: these emerged as the key enablers of transformation processes in all of the cases above. In fact, our research shines a light on how the central key dimensions of digital transformation are often mediated by a sixth - the data strategy - which in this sense functions as an intermediate level between formalizing strategy and aligning IT.
In any case, there is one fundamental point to consider. The objective of these paths is often to expand business models by constructing an all-encompassing view of customers and/or processes. But this vision comes up against so much complexity, both in terms of technological systems and organizational structures, that a number of intermediate stages of formalization and data utilization emerge. More advanced stages can virtually enable more ambitious applications, but this doesn’t mean that it is always desirable to do so, because these ambitions have to contend with the complexity involved in building and managing enabling conditions.
This study highlights three typical approaches to data-enabled evolutionary pathways.
The first (elaborate) focuses on data-enabling a traditional business model for the company in question. The use of data here is limited, and occurs for the most part in individual functions, with little coordination in terms of transformative objectives or technological systems.
The second (extend) is found in companies that have adopted a data strategy, which leads to a clear digital evolution in the business model. Systems have been created that make it possible to integrate existing data and recently-acquired data, with an eye to extending the traditional business model.
The third (explore) calls for continual experimentation. Here the company relies on a technological base and a solid organizational structure, which make it possible to monitor the environment, conduct continuous tests and experiments, and then integrate the results into the operational model and the business model. The more technologically advanced organizations are the ones that lean toward this approach. The company learns from data incrementally and, enabled by the corporate culture, technological systems, and organizational structures, generally assumes a more fluid mentality, gradually adopting more evolved business models.
Generally speaking, if we think in terms of aligning the six dimensions of digital transformation, and utilize this framework to read and interpret initiatives that are ongoing or under consideration, this can help us focus more squarely on indispensable elements that can facilitate the digital transition the company aspires to, favoring an informed, pragmatic approach to achieving related objectives.