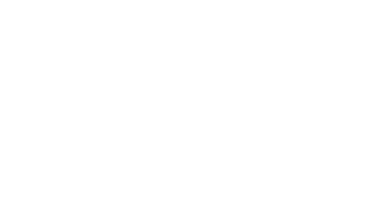
- Start Date
- Duration
- Format
- Language
- 14 Apr 2025
- 4,5 days
- Class
- Italian
Gestire in modo integrato e coerente una rete di vendita potenziando la capacità di prendere decisioni efficaci e sviluppando una visione strategica del sales management.
Family influence on companies goes far beyond mere equity stakes. Leveraging advanced machine learning tools, such as ChatGPT, we observed that families maintain a subtle yet significant control over firms, even in cases where ownership percentages are small. This fresh perspective provides key insights for investors and managers and highlights the importance of adopting a broader definition of a family firm, based not only on ownership, but also on management, values, and legacy.
Historically, the classification of family firms has been based on equity ownership, with previous studies primarily relying on specific thresholds of voting rights or shares held by family members. However, this approach often oversimplified the complexity of family involvement, failing to capture the nuances of influence, particularly in cases where families retained control through governance roles or cultural legacy, despite lower ownership stakes.
Working with Isabelle Le Breton-Miller, Danny Miller, Valerio Pelucco, and Marc Van Essen, we found at least 30 definitions of a family firm in scholarly literature. However, most measurements are based on quantitative, readily available data, i.e. on ownership.
We sought to address these gaps with the help of an emerging technology capable of transforming texts into data: machine learning (ML). Our research questions centered on how to measure family influence on a company and whether non-traditional indicators, such as management, family values, and legacy, could provide deeper insights into what it means for a company to be a “family firm.”
To explore these questions, we utilized ChatGPT, an ML application capable of processing vast datasets and identifying patterns of family influence based on factors beyond ownership. Since OpenAI, the company that owns ChatGPT, claims that the version we used was trained on around one-third of all Internet content, we were confident it was exposed to the most salient information about listed companies.
With simple prompts, we asked ChatGPT to rate the overall “familiness” of 3,864 listed firms across 43 countries and to compute sub-scores of the “familiness” of the ownership structure, management structure, company values, and legacy. Legacy is an intangible force that affects the decision-making of the next generation. The concept of family legacy, encapsulated as the “intention for transgenerational control,” constitutes a central preoccupation for many family entrepreneurs.
By running every prompt 30 times, we believe we shielded ourselves from the risk of ChatGPT’s “hallucinations” (answers not rooted in the training set). The answers turned out to be very consistent.
In most cases, the outcome of our method matched the classification of widely used databases. When it did not, further investigation revealed, in more than 90% of the cases, that ChatGPT’s judgment was more accurate.
Thanks to our research, we were able to create a more nuanced picture of family firms. In countries like Japan, where families often have limited equity but significant cultural influence, ChatGPT identified more family firms than traditional equity criteria.
For managers, understanding the cultural and emotional priorities of family-controlled businesses(such as preserving long-term control and family legacy) can provide a strategic advantage. Such is the case with M&As: If it’s true that cultural similarity is a component of a merger’s success, identifying family firms more accurately can help managers identify the best matches for such operations.
Financially, our study suggests that classifying family firms based on these broader criteria can yield valuable information for investors, offering potential insights into stock returns and corporate governance. We observed that family firm portfolios based on our definition perform better than portfolios based on traditional definitions.
Our findings have relevant implications for research. By expanding the definition of family firms to include legacy, governance, and values, we open new avenues for studying the behavior and performance of these firms, potentially offering them better advice.
Mario Daniele Amore, Valentino D’Angelo, Isabelle Le Breton-Miller, Danny Miller, Valerio Pelucco, Marc Van Essen. “Using machine learning to identify and measure the family influence on companies.” Journal of Family Business Strategy, 2024, 100644.
DOI: https://doi.org/10.1016/j.jfbs.2024.100644.